A New Metric to Evaluate Auditory Attention Detection Performance Based on a Markov Chain
Simon Geirnaert, Tom Francart, Alexander Bertrand
November, 2019
Abstract
Auditory attention detection (AAD) is an essential building block for future generations of neuro-steered hearing prostheses. In a multi-speaker scenario, it uses neural recordings to detect to which speaker the listener is attending and assists as such the noise reduction algorithm within the hearing device. Recently, a multitude of these AAD algorithms has been developed, based on electroencephalography (EEG) recordings. With the emergence of AAD algorithms, a standardized way of evaluating these AAD algorithms becomes paramount. However, this is not trivial due to an inherent trade-off between detection delay and accuracy. We propose a new performance metric to evaluate AAD algorithms that resolves this trade-off: the expected switch duration (ESD). The ESD is based on a Markov chain model of an adaptive gain control system in a hearing aid and quantifies the expected time needed to switch its operation from one speaker to another when the attention is switched. We validate the metric on simulated data and show on real EEG recordings that it is an interpretable metric that allows fair comparison between AAD algorithms, combining both the accuracy of the AAD algorithm and the time needed to make a decision.
Publication
In Proceedings of the 2019 27th European Signal Processing Conference (EUSIPCO), A Coruña, Spain, pp. 1-5, Sept. 2019
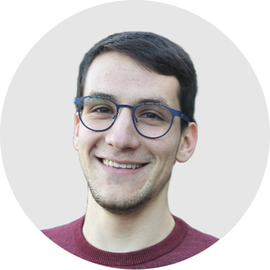
Postdoctoral researcher
My research interests include signal processing algorithm design for multi-channel biomedical sensor arrays (e.g., electroencephalography) with applications in attention decoding for brain-computer interfaces.