Electroencephalography-Based Auditory Attention Decoding: Toward Neurosteered Hearing Devices
Simon Geirnaert, Servaas Vandecappelle, Emina Alickovic, Alain de Cheveigné, Edmund Lalor, Bernd T. Meyer, Sina Miran, Tom Francart, Alexander Bertrand
June, 2021
Abstract
People suffering from hearing impairment often have difficulties participating in conversations in so-called cocktail party scenarios where multiple individuals are simultaneously talking. Although advanced algorithms exist to suppress background noise in these situations, a hearing device also needs information about which speaker a user actually aims to attend to. The voice of the correct (attended) speaker can then be enhanced through this information, and all other speakers can be treated as background noise. Recent neuroscientific advances have shown that it is possible to determine the focus of auditory attention through noninvasive neurorecording techniques, such as electroencephalography (EEG). Based on these insights, a multitude of auditory attention decoding (AAD) algorithms has been proposed, which could, combined with appropriate speaker separation algorithms and miniaturized EEG sensors, lead to so-called neurosteered hearing devices. In this article, we provide a broad review and a statistically grounded comparative study of EEG-based AAD algorithms and address the main signal processing challenges in this field.
Publication
IEEE Signal Processing Magazine, vol. 38, no. 4, pp. 89-102, 2021
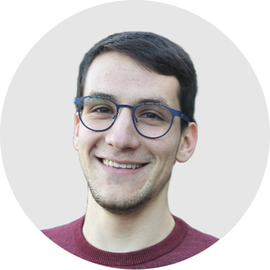
Postdoctoral researcher
My research interests include signal processing algorithm design for multi-channel biomedical sensor arrays (e.g., electroencephalography) with applications in attention decoding for brain-computer interfaces.